Smartheart 2022-2023 Project – Deep Learning-Based Analysis of 12-LEAD Electrocardiograms for Cardiovascular Disease Risk Prevention
Evan Wen (Form VI), JP Salvatore (Form VI), Max Liu (Form VI), Alan Zhong (Form V)
Project Summary + Project Goal
Cardiovascular disease is the leading cause of death worldwide which is often diagnosed with electrocardiograms (ECG). The electrocardiogram is a non-invasive test which measures the electrical signal of the heart via electrodes placed on the chest and limbs. Diagnosing ECGs was originally a task left to medical specialists, but in recent years AI has emerged as an approach to automate the diagnosis. However, there are limitations to the diagnosis leading to various inaccuracies AI has been unable to fully conquer to this date. Currently, our project is focused on attempting to solidify this approach with optimal diagnosis results.
Current State
In order to denoise our data, we explore various signal processing methods, such as finite impulse response, discrete wavelet transforms, feature engineering, and fourier transform. Using time-series classification networks, we then calculate probabilities for each diagnosis. Specifically, we test LSTM, RNN, and transformer models due to their popularity within natural language processing. We also experiment with popular image-classification models, such as the vision transformer on spectrograms generated from the waveform. Our results are evaluated against other approaches from the 2020 George B. Moody PhysioNet Challenge. The overarching research question is whether we can accurately assess a patient’s risk level for future disease. If diagnosed early enough, cardiovascular disease is curable. However, current work has remained focused on diagnosing patients already compromised by their condition. We also examine the feasibility of developing tools to calculate the probabilities for the 27 most common cardiovascular diseases. To achieve this, we seek to analyze discrepancies in healthy ECGs as well as personalized patient data in conjunction with our current work.
Next Steps
We are doing a literature review of different algorithms that have achieved high scores in the 2020 PhysioNet Challenge. We will implement promising approaches and improve on them by using new signal preprocessing methods. If multiple preprocessing methods achieve good results, we may combine them to create an ensemble classifier. Our goal is to create a model that achieves a competitive score based on the PhysioNet Challenge scoring metric. We also plan on developing a computer vision algorithm to scan ECGs that we have kindly received from Dr. Robert Masci, chairman of the cardiology department at the Newton Medical Center.
Research Week Poster
Meet the Team
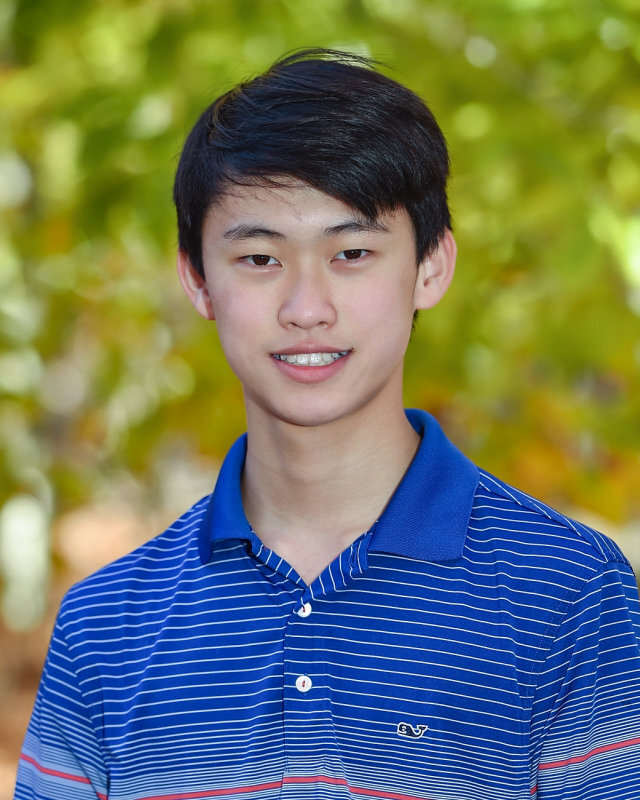
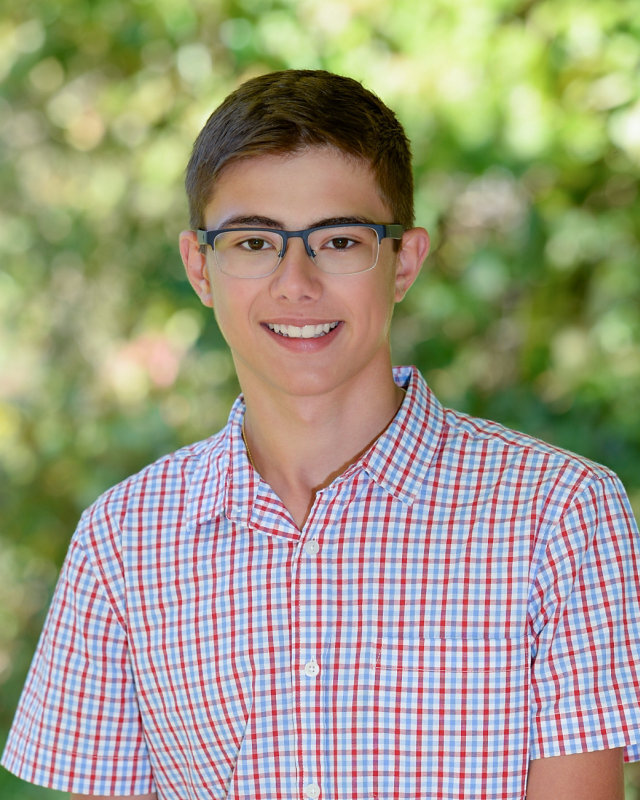
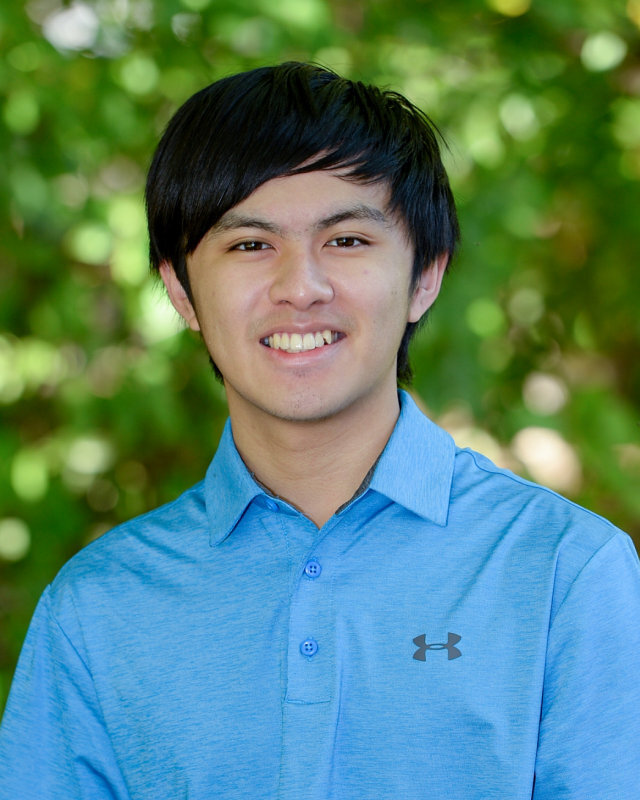
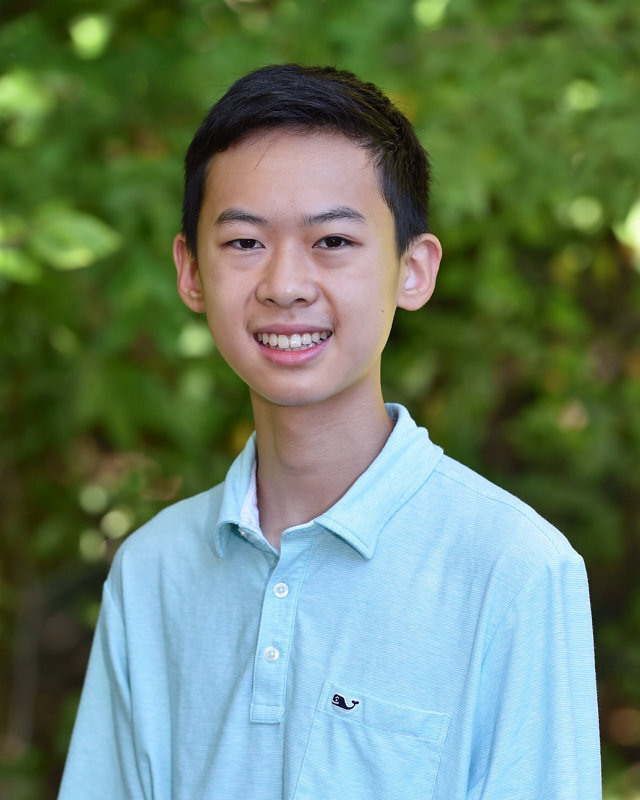
Evan Wen
JP Salvatore
Max Lui
Alan Zhong
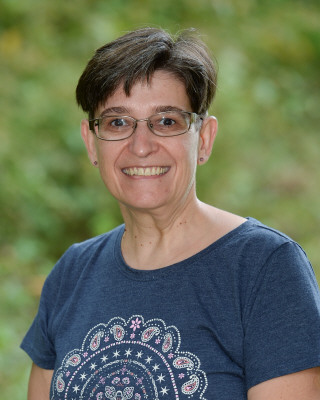